Emerging Computational Tools for Predicting Mixing of Bulk Solids
March 6, 2014
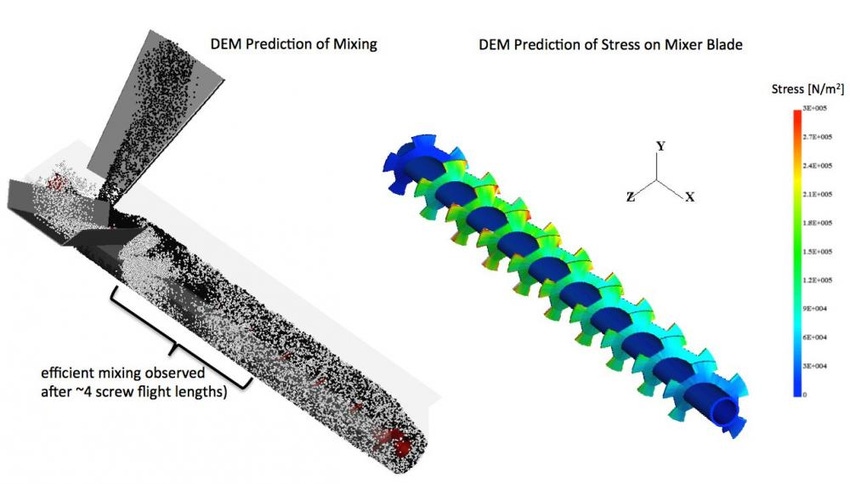
Mixing of bulk solids is a critical operation in many manufacturing processes. One commonly encountered problem is a blender’s poor performance caused either by a variation of the powder material properties or operating conditions. A link between these independent variables in the process to critical quality attributes, often termed as the design space, are usually not known a priori. An understanding of the mixing mechanisms and the effect of operating conditions on the final blend can help to establish the design space that could prevent costly equipment replacement. Although having an understanding of the design space can predict mixing efficiency and improve the product quality, it is not a trivial task to perform. One challenge is the large costs associated with performing large number of experiments that include variation of material properties, operating conditions, and blender geometries. However, with computers becoming faster and with emerging mathematical and computational models, the number of experiments can be significantly reduced or even eliminated in some cases. Furthermore, quantities not easily measured in the experiments can be predicted using a computer model.
The discrete element method (DEM) is a computation tool that has found wide applicability in many applications where bulk materials are processed and handled. It is a numerical technique and the theoretical basis of the method is from Sir Isaac Newton’s laws of motion. The inputs to a DEM model of a mixing process will typically include the blender geometry and information such as particle shape and size distribution, particle density, and friction. Forces such as cohesion, electrostatics, and fluid drag can also be included. The motion and interaction of every particle in the system is tracked over a period of time. Researchers are also investigating more advanced techniques such as coupled DEM-CFD (computation fluid dynamics) and DEM-PBM (Population Balance Methods) to predict mixing and blending.
A wealth of information, such as mixing flow patterns, mixing rates, impact and abrasive forces on blades, power consumption, wear patterns on surfaces, attrition rates, and residence time of particles can be obtained from these models.
See Fig. 1 (attached)
For example, Fig. 1 shows DEM predictions of mixing of two materials coming down transfer chutes into a continuous screw mixer. It is seen that the mixing is achieved after ~ 4 screw flight lengths and the material does not show any de-mixing or over- blending after that. In addition, the stress patterns (and thus wear patterns) on the mixer blade are also seen.
Although DEM is emerging as a powerful tool to study bulk materials, there still exists significant limitations and common misconceptions. The primary limitation is its inherent computational intensity. Since the technique tracks each individual particle and its interactions over time, large numbers of particles in the system results in practically restrictive computational times. In addition, representation of particle shapes and their inherent contact detection algorithms are computationally intensive and time consuming. However, these cannot be ignored because studies have shown that mixing and segregation predictions in blenders using DEM are sensitive to mechanical interlocking arising due to the representation of particle shape in the model. The advent of faster computers will eventually pave the way for overcoming some of these major limitations. It is also noteworthy to mention that a common misconception is that DEM is a plug-play tool. However it is far from it and it is critical to understand the sensitivity of the model inputs on the DEM outputs. In other words, garbage-in is equal to garbage-out from the model, and it is imperative to not only understand the critical inputs but also validate the results to gain increased confidence in future predictions.
Dr. Rahul Bharadwaj is a senior R&D engineer at Jenike & Johanson Inc. He has nearly a decade of experience in the development, validation, and application of computational tools such as Discrete Element Modeling (DEM), Computational Fluid Dynamics, and Finite Element Analyses. He received his M.S. in Mechanical Engineering from the University of Kentucky, and a Ph. D. in Mechanical Engineering from Purdue University.
For related articles, equipment reviews, and news, visit our Mixers & Blenders Equipment Zone
Click here for information about the International Powder & Bulk Solids Conference & Exhibition
You May Also Like