Predicting Blending Behavior through Dynamic Powder Characterization
April 20, 2017
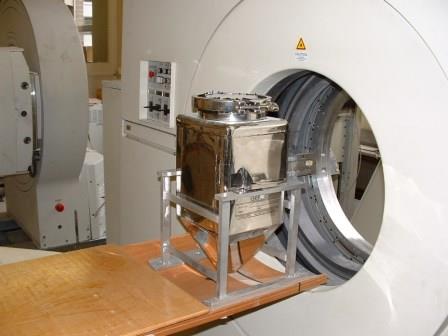
In industries as diverse as cosmetics, pharmaceuticals, food production, and metal powder processing, homogeneity is crucial to the quality of a finished product. Whether it is the mechanical properties of a sintered metal component, the uniform dispersion of an active ingredient in a drug, or achieving consistent flavor, fragrance, or color, achieving blend uniformity is a critical step in the manufacturing process.
This article considers the tools available to assess blending performance, including research carried out using positron emission tomography (PET), and introduces the concept of dynamic powder testing. Data is presented showing the correlation between dynamic flow and PET data – even when unexpected behavior is observed – demonstrating how dynamic flow data can be used as a reliable predictor of blending behavior and applied to develop and optimize blending processes.
The Knowledgebase Underpinning Optimized Blending
Individual powders can be difficult to characterize reliably, so predicting the behavior of a multi-component mixture in a blender – a critical issue for formulation development and optimization – represents a significant challenge. Powder characterization techniques must improve the understanding of blending processes, promote the selection of appropriate feed materials (where there is choice), and streamline scale-up.
Click here for information about the PBS Toronto event, May 16-18, 2017
A complementary requirement is for techniques that enable continuous monitoring of blenders within the plant environment, as opposed to the more common practice of thief sampling 10-30 samples from different points within the blender for analysis in the laboratory. Although widely used during development and into manufacturing, this approach has three major drawbacks: the sampling process itself disturbs the bed; a very large number of samples are needed to generate an assessment of homogeneity; and it is a time-consuming and lengthy process not conducive to effective decision making in the manufacturing environment.
Increasing interest in continuous, on-line monitoring has seen the adoption of spectroscopic techniques (Near Infrared and Raman) and other methods, such as Laser Induced Fluorescence (LIF) and thermal effusivity. However, they are usually limited to a single point measurement that can compromise their ability to support full optimization. It is usual to retain QC back-up to provide an assurance of success and the issue of method validation, usually by thief sampling, remains.
Investigating Blending Behavior with Positron Emission Tomography
Positron emission tomography (PET) is a non-invasive imaging technique that detects gamma rays released by a positron-emitting tracer, and is capable of characterizing an entire blended mass through the wall of a container1. The method can therefore be used to evaluate the effect of process parameters on the blending process without affecting either the contents of the blender or the structure of the powder bed, thereby providing a robust assessment of the state of blend without disturbance. PET delivers reliable data that can be correlated with off-line test data to identify parameters that are relevant to the blending performance.
Figure 1: Assessing homogeneity of a blend using a PET camera
Figure 1 shows a bin entering the PET camera to measure the extent of homogeneity. The bin contains microcrystalline cellulose (MCC), with a small bolus (2% by mass) containing radioactive tracer. After being tumbled for a pre-determined number of revolutions, the bin is transferred to the camera to evaluate the degree of dispersion of the radioactive particles. It is then returned to the blender and the sequence is repeated to the point of acceptable homogeneity.
PET enables detailed scrutiny of the blended mass in a way that delivers a novel insight into blending. The light intensity in each region of the image relates directly to the number of radioactive counts emanating from it, i.e. the amount of tracer present, and each scan produces approximately 106 data points each representing just 43 cu mm. This high resolution and sensitivity enables the tracking of low concentrations of tracer and detailed examination of areas where poor mixing is suspected. However, PET’s dependence on the use of a radioactive tracer limits it application to the laboratory, only one component can be tracked, and analysis is relatively slow, although comparable to the rates achieved with thief sampling.
Correlating PET Analysis with Powder Flow Measurements
A series of experiments were conducted to assess the impact of process variables on blending performance and to identify powder properties that rationalized observed behavior. The PET data relating to blend homogeneity are presented as a %RSD, with lower values associated with greater homogeneity. Below 5% the blend can be considered effectively homogeneous.
When a 2% radioactive bolus of microcrystalline cellulose (MCC) was blended into a five-liter volume of MCC, higher blender speeds resulted in faster blending, an outcome consistent with expectations (figure 3).
Figure 3: Higher blender speeds lead to faster blending with MCC; fewer revolutions are required to reach homogeneity.
However, repeating the experiment with sodium benzoate produced opposing results, with lower blender rotation speeds accelerating the blending process (see figure 4).
Figure 4: With sodium benzoate, higher blender speeds are detrimental to processing performance; more revolutions are required to achieve blend homogeneity.
Both MCC and sodium benzoate were characterized using a number of powder testing techniques to determine whether any measurable powder properties could predict this observed behavior.
Dynamic powder characterization involves measuring the axial force and torque acting on a twisted blade as it rotates through a sample along a defined helical path. The measurements are used to determine a value for flow energy which describes the powder’s resistance to flow. The powder’s response to varying flow or shear rates slowly is quantified by changing the speed of the blade and is directly relevant in assessing the impact of blender speed. Many powders exhibit non-Newtonian behavior and flow more easily at higher flow rates, i.e. flow energy reduces with increasing blade tip speed. MCC exhibits this behavior but for sodium benzoate, flow energy increases as blade tip speed increases (Figure 5). These data directly correlate with the trends observed in PET analysis of the blending performance.
Figure 5: Dynamic tests show that MCC flows more easily at higher flow rates, while sodium benzoate exhibits the opposite trend.
Morphologically, sodium benzoate and MCC are dissimilar. The spherical MCC particles become more aerated with increasing blender/blade tip speeds so they disperse and mix more easily. The sodium benzoate particles are platelet shaped, locking together more enthusiastically at higher flow rates and experiencing translational/rotational frustration which inhibits effective dispersion. The other traditional tests undertaken on this powder did not identify this behavior.
Multi-Component Blends
In a further experiment, a 2% bolus of radioactive MCC was loaded on top of a layer of two liters of MCC, layered on top of three liters of lactose. Figure 6 shows the data generated from analysis with the PET camera and from dynamic characterization tests for lactose, MCC, and the binary mixture.
Figure 6: Dynamic flow energy data for a mixture of lactose and MCC predicts that MCC will blend less easily into a lactose/MCC mixture than into either individual component.
These results show that the tracer MCC blends more easily into bulk MCC than it does into an identical volume of lactose. Also, that while it might be expected that MCC/lactose mixture results would lie somewhere between these extremes, the mixture is associated with the worst blending performance. The flow energy values suggest that the poor dispersion is linked directly to an increased resistance to flow exhibited by the MCC/lactose mixture, which inhibits particle movement and dispersion.
Although techniques such as PET are clearly some way from being an accessible tool for industry they deliver value by facilitating the identification of readily available characterization methods that can be used to optimize blending processes.
The experimental results robustly reinforce the correlation between flow energy and blending time, and demonstrate that dynamic powder characterization can accurately predict blending performance. Comprehensive and relevant powder testing techniques can play an important role in reducing the dependence on expensive, large scale blending trials.
Jamie Clayton is operations director, Freeman Technology. For more information, visit www.freemantech.co.uk.
References
[1] Seville, J. P. K., Parker, D. J. & Ingram, A., 2005, “Probing Processes Using Positrons”, Chemical Engineering Research & Design, 83, A7, pp 788-793
[2] Freeman, R. E., 2007, “Measuring the flow properties of consolidated, conditioned and aerated powders – A comparative study using a powder rheometer and a rotational shear cell”, Powder Technology, 174, 1-2, pp 25-33
You May Also Like