Improving Dryer Yield with Intelligence
August 24, 2017
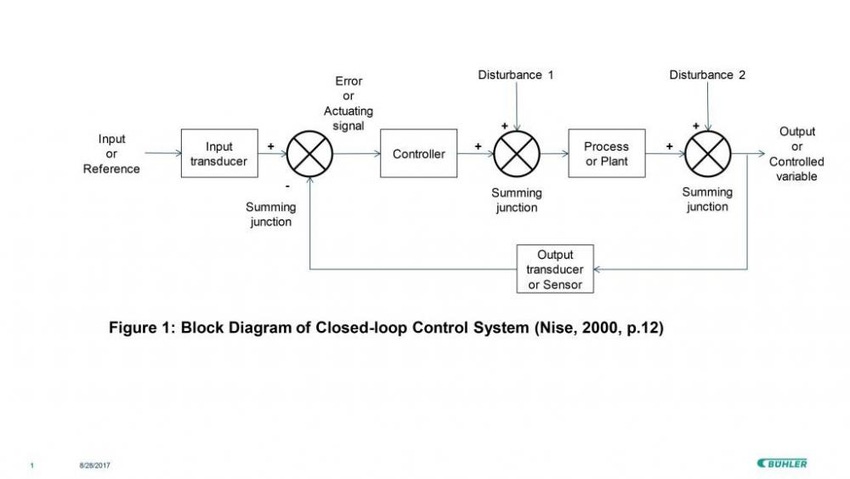
Controlled drying parameters help to ensure that a food or feed product is shelf-stable. However, one single processing deviation can dramatically affect batch results and yield. This may be a result of over drying due to unnoticed process shifts. Today, many food and feed factories are becoming leaner and operations staff has less time to closely monitor dryer performance.
Over drying, or drying to a water content far below a reasonable shelf stable level, is unfortunately a common practice throughout the food and feed industries. Over drying wastes energy and increases a manufacturer’s carbon footprint. It can also lead to undesirable product characteristics, such as an inconsistent color or texture. When product is over dried it will take a longer time to cool to a packable temperature.
As a result, being able to efficiently control moisture is vitally important for any dryer operation. Intelligent dryer control systems, coupled with cloud based data storage and analytics, has the capability to solve this complex problem.
Intelligent Dryer Control – System Design
First, it is important to systematically consider the appropriate method of control before moving into sensor selection, electronics architecture, and data processing. Having a strategic, desired end result is also critical to effectively control moisture in a dryer and improving yield. For example, an objective could be to control moisture to a target value within one half retention time of the dryer with no more than 1% wwb high deviation. Learning from Control Systems Engineering, “top-down design, a high-level picture of the requirements is first formulated; then the function and hardware required to implement the system are determined.” (Nise, 2000, p.9).
Depending on the application, a processor can choose an open-loop or closed-loop controller. In open loop control, a simple factor could be adjusted (e.g. time or temperature) in a fixed way by product specifications (e.g. simple recipe control). In closed loop control, discharged product can be continuously monitored, using sensors to gather moisture data.
For the basis of this article, a closed loop controller will be considered for moisture management. Following is a basic block diagram that demonstrates a closed loop controller.
Schematic modelling, development of a transfer function or state-space representation is important to mathematically relate inputs to outputs. This relationship is the root of a controller design which then allows for selection of input and output devices. It is also a basis for confirmation testing of prototype systems.
Selecting Moisture Sensors
Sourcing the appropriate technology to measure water content, that will ultimately provide valuable feedback to a dryer’s control system, is an important consideration when adding an intelligent system to manage yield losses. Following are key questions to consider when selecting a sensor:
• Shape/Size/Density – Does your food have moisture gradients at the sensing location? For example, is the surface significantly dryer than the inside of the product?
• Number of products – How does the sensor react to various product types, product families? How are adjustments accommodated for these product changes?
• Ease of use – How is the sensor setup? How does the sensor integrate within existing control architecture? How are reference measures input? What correction factors are considered (e.g. density/temperature/volume of reference)?
• Food safety – Can this sensor be in contact with food? If yes, how easy it is to clean? Is it suitable for the environment which it shall be exposed to?
• Accuracy/Repeatability – How accurate will the moisture reading be against a known moisture level to provide valid feedback to a controller?
• Price – What is the cost for the sensor up-front? (Beware: A typical purchase price is usually a lowest cost factor) What is the cost for maintenance and long term use? What is the cost for implementation?
Clearly, there is no one path for every product. Sensor selection for a process and a facility are unique. A cross functional team of process engineers, maintenance engineers and quality assurance should be assembled to assess and ultimately determine the correct product sensor.
Connected Architecture
Once a sensor has been selected, the next step is determining how this feedback device will be incorporated to an existing dryer control. Factors such as signal exchange, communications protocols, data collection and storage should be considered. Typically, sensing equipment will range from simple (single channel analog feedback = sensed moisture content) to complex sensor + analyzer combinations, providing a mix of sensed and soft data for further use. Some sensor + analyzer systems also allow for two-way communications where key parameters can be sent to the moisture sensing system for a deeper level of integration into existing control systems. Latest technology can provide a deep insight into middle-state products which if controlled can significantly improve yields and reduce waste by catching process deviations before they reach the bag.
Understanding the automation technical specifications and barriers for incorporation to your system is as important to success as selecting the correct sensing technology for your product.
Cloud Storage and Analytics
The drying process, in some applications, is extremely slow. The effect of adjustments made by intelligent controls can take many hours to be revealed in a form of on or off specification product quality. Data acquisition systems allow for near real-time uptake and analysis of key process indicators (KPI) to gain a view inside the process as changes take effect. An existing infrastructure may not have the “computing horsepower” to handle and analyze different amounts of data. With the continual decline in data storage infrastructure costs, rise of security in internet-based (cloud) solutions, and declines in overhead costs, many are turning to externally-sourced data management solutions. This is an alternative to a legacy-on-premise infrastructure that requires supporting technical know-how, obsolescence management, and risk-mitigation strategy to ensure storage is maintained throughout the life span of the equipment. Other advantages of cloud-based architecture allow for improved risk mitigation as many mainstream providers of cloud have superior data backup, and disaster recovery mitigation plans in comparison to self-managed solutions.
Analytical applications are widely available to complement many cloud-based data repositories. This infrastructure allows for many machines to push key process data to co-located data sites for simultaneous processing. This co-location allows for use-based processing which can significantly lower costs compared to on premise dedicated infrastructure basis. Processing of key data in the cloud for multiple machines allows for an easy comparison of OEE, efficiency, capacity, energy, and many other machinery measures. This can provide insights on which machines are best-in-class. It can also provide a clear return on investment (ROI) basis for others whom cannot make the grade.
Long-Term Considerations
With a control strategy and architecture in mind, combined with a correctly selected sensor network, it’s important to consider the long-term aspects of your new investment plan. ROI analysis is only as good as the possibility of realizing such gains. Miscalculations in the long-term maintenance requirements of keeping these systems at high-performance levels can quickly overshadow gains. A few areas of consideration:
• Calibration – How often and at what cost does each new sensor require calibration services? What amount of scrap product is produced during calibration process?
• Tuning – What tuning procedures are required for each added control loop? Is customized tuning required for each set of processing conditions to gain control quickly, or does one tune fit all?
• General Maintenance – What is the failure rate of newly added equipment? Will it fail before ROI is realized? What is the cost of general servicing frequency of new equipment?
• Know-how related – What staff is required to maintain a specialty control? Who knows the details of such system and what time investment is required from this staff to continue to hold this system in top performance?
• Virtual infrastructure – What are costs related to ongoing maintenance and support of cloud based utilities? What does an incremental cost increase do to savings rates?
Using the factors above in combination with your own considerations, recalculate your ROI plan to ensure a sustainable plan can be implemented. Leading machinery OEMs understand these pains and are investing effort in providing infrastructure and know-how support services to support your long term savings concept.
Long-Term Pet Food Study
In early Q1 2017, Bühler Aeroglide installed a moisture control system aimed at closed-loop management of product moisture content in a U.S. pet food processor’s facility. Bühler’s experts paired this system with a new AeroDry two-pass dryer equipped with the company’s latest dryer management software. Utilizing cloud infrastructure, Bühler’s team began monitoring operator-based moisture control, which is typical in the industry today. With legacy operator-based control, moisture levels averaged 4.2% wwb with a standard deviation of 2.4. With the addition of the new closed-loop moisture control system and three months of OEM-based support, average moisture increased to 6.4% wwb with a standard deviation of 1.6. Bag guarantees for this product is about 10% wwb, therefore there are further gains possible. Without the cloud storage and analytics technology, quantification of these initial savings would have gone underappreciated. Overall, the proof of concept is the ability to sustainably raise moisture and decrease deviation which equates to improved yields and reduced energy.
The case study provides a template for intelligent control systems, providing tangible improvements to the food supply chain. The case study client is reaping the rewards of improved process control and improved dryer production rates. Furthermore, accessibility to its data and summaries of KPIs are available utilizing cloud-based technology. This top-down approach can be transferred to your moisture management project and hopefully bring your KPIs to levels not previously achievable.
Doug Beloskur is product manager automation at Bühler Aeroglide. For more information, visit www.buhlergroup.com.
References
1. Nise, Norman S. (2000). Control Systems Engineering, 3rd edition. New York, NY, John Wiley & Sons Inc.
For related articles, news, and equipment reviews, visit our Drying & Thermal Solids Processing Equipment Zone
Click here for a List of Drying & Thermal Solids Processing Equipment Manufacturers
You May Also Like